Learning tactile skills with FingerVision
Master's thesis
Role: Robotics&Data Scientist
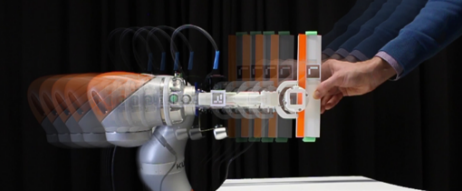
Abstract: Assembly tasks require rich feedback that enables tracking and adjustment of task execution parameters to unforeseen changes in the environment. Due to recent breakthroughs in computer vision, many architectural systems use video and depth cameras to guide assembly through sensory feedback. However, visual feedback alone is insufficient for performing manipulation tasks that crucially depend on contact with external objects and require force estimation at contact points. Although conventional force-torque sensors can be used to extend robot capabilities in this direction, they tend to be expensive.
In this thesis, we investigate the feasibility of employing an inexpensive vision-based alternative in closed-loop control scenarios – a variant of the FingerVision sensor that leverages the advances in computer vision to compensate for the lack of problem-specific hardware. We develop and evaluate a range of feedback controllers that use various modalities provided by the FingerVision sensor as input. In particular, by combining tactile feedback with real-time gripper and robot control algorithms, we demonstrate grasp adaptation, object shape and texture estimation, slip and contact detection, force and torque estimation. Furthermore, we show that the data delivered by the sensor is of sufficient quality to enable learning of auxiliary tactile skills – mapping directly from contact sensations to the force applied at an object in contact and to the viscosity and granularity of a substance being stirred by means of a spoon. Finally, the proposed tactile feedback controllers and learned skills are combined together to demonstrate applicability and utility of tactile sensing in collaborative human-robot architectural assembly tasks